
Welcome to DitecT (Data & innovative technology-driven Transportation) Lab!
​
Large IT corporations such as Google’s entry into the car market and the rapid growth of Uber and Didi/Kuaidi marks a new era for the "next-generation transportation systems". Transportation infrastructure, vehicle fleets, and people’s driving and travel behavior would undergo major transformations. Understanding such changes requires knowledge in optimization, statistics, machine learning, while domain knowledge in transportation systems continues to play a pivotal role. This is a unique and exciting time to study and conduct research on transportation science from an interdisciplinary perspective.
Mission: DitecT Lab aims to collect, analyze, and discover travel behavior patterns from the big data using various types of traffic sensors including individual tracing devices, and uses such information to guide us in modeling and forecasting the changes occurring in radically transformed transportation systems. Our ultimate goal is to help decision-makers to understand the upcoming changes and prepare them for effective planning and management of next generation transportation systems.
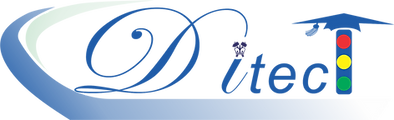
RESEARCH
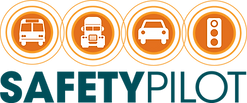

Clustering Travelers Based On Travel Activity Patterns Using Michigan’s Connected Vehicles Trajectories
People travel for the sake of accomplishing activities. Therefore travel demand is a derived demand. Understanding the travel demand level is a critical step for the classical four-step travel forecasting model. However, the four-step model predicts travel demands at an aggregate level which shadows the behavioral motivation of traveling. With the popularity of ubiquitous sensors, such as GPS devices, smartphones, and connected vehicles, individuals can be traced in terms of where they go at what time and what activity they may perform. One notable data to this purpose is collected through the world's first and largest connected vehicle testbed - Safety Pilot project. Please refer to this set of slides for a brief description of the data format.
Based upon this information, can we infer the population travel demand level in a region from trajectories of a portion of travelers? Answering this question requires machine learning techniques. Such research will provide an empirical ground for activity-based travel demand modeling.
Travel Behavior Modeling Incorporating Shared Mobility
​​
Shared mobility, including ridesharing, car sharing, and ride-sourcing is becoming increasingly popular in recent years, thanks to the cutting-edge technologies in sensing, computing, and communication (e.g., connected and automated vehicles). Many new research questions await to be answered:
-
Will people travel the same way as they do now when shared mobility becomes a pivotal part of transportation systems?
-
As a new travel mode, how does ridesharing change traffic patterns? How can building high-occupancy toll (HOT) lanes influence people's travel behavior?
-
Does the emergence of Transportation Network Companies (TNC) like Uber or Lyft worsen or relieve traffic congestion?
-
Will the sharing of autonomous vehicles transform the way people choose travel modes and routes?
​
Solutions to these questions are not trivial, which requires a transformative framework for traffic equilibrium.

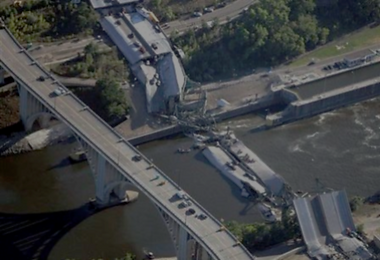
Systems Resilience & Travel Behavior Under Disrupted Networks
​
Network disruption occurs due to either natural disasters (e.g., hurricane) or man-made activities (e.g., terrorist, structural failure).
I am interested in understanding how travelers react to transportation network disruption (which is on the demand side) and how resilient infrastructure should be designed in the planning, mitigation, or restoration stage (which is on the supply side).
​
One such application was my doctoral dissertation about modeling travelers' route choice behavior after the collapse of the I-35W Bridge and restoration. Another application is an on-going research, funded by NSF, to understand how travelers in Austin, Texas change their travel mode choice after Uber/Lyft discontinued their service.